92,95 €
92,95 €
inkl. MwSt.
Sofort per Download lieferbar
46 °P sammeln
92,95 €
Als Download kaufen
92,95 €
inkl. MwSt.
Sofort per Download lieferbar
46 °P sammeln
Jetzt verschenken
Alle Infos zum eBook verschenken
92,95 €
inkl. MwSt.
Sofort per Download lieferbar
Alle Infos zum eBook verschenken
46 °P sammeln
- Format: PDF
- Merkliste
- Auf die Merkliste
- Bewerten Bewerten
- Teilen
- Produkt teilen
- Produkterinnerung
- Produkterinnerung
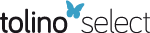
Bitte loggen Sie sich zunächst in Ihr Kundenkonto ein oder registrieren Sie sich bei
bücher.de, um das eBook-Abo tolino select nutzen zu können.
Hier können Sie sich einloggen
Hier können Sie sich einloggen
Sie sind bereits eingeloggt. Klicken Sie auf 2. tolino select Abo, um fortzufahren.
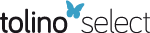
Bitte loggen Sie sich zunächst in Ihr Kundenkonto ein oder registrieren Sie sich bei bücher.de, um das eBook-Abo tolino select nutzen zu können.
This textbook is designed for an undergraduate course in data science that emphasizes topics in both statistics and computer science.
- Geräte: PC
- mit Kopierschutz
- eBook Hilfe
Andere Kunden interessierten sich auch für
- Dirk P. KroeseData Science and Machine Learning (eBook, PDF)92,95 €
- Darrin SpeegleProbability, Statistics, and Data (eBook, PDF)92,95 €
- Basilio de Braganca PereiraStatistical Learning Using Neural Networks (eBook, PDF)55,95 €
- Julian J. FarawayLinear Models with Python (eBook, PDF)90,95 €
- Przemyslaw BiecekExplanatory Model Analysis (eBook, PDF)51,95 €
- Bing LiSufficient Dimension Reduction (eBook, PDF)51,95 €
- Luca ScruccaModel-Based Clustering, Classification, and Density Estimation Using mclust in R (eBook, PDF)57,95 €
-
-
-
This textbook is designed for an undergraduate course in data science that emphasizes topics in both statistics and computer science.
Dieser Download kann aus rechtlichen Gründen nur mit Rechnungsadresse in A, B, BG, CY, CZ, D, DK, EW, E, FIN, F, GR, HR, H, IRL, I, LT, L, LR, M, NL, PL, P, R, S, SLO, SK ausgeliefert werden.
Produktdetails
- Produktdetails
- Verlag: Taylor & Francis eBooks
- Seitenzahl: 650
- Erscheinungstermin: 13. April 2021
- Englisch
- ISBN-13: 9780429577505
- Artikelnr.: 61377195
- Verlag: Taylor & Francis eBooks
- Seitenzahl: 650
- Erscheinungstermin: 13. April 2021
- Englisch
- ISBN-13: 9780429577505
- Artikelnr.: 61377195
- Herstellerkennzeichnung Die Herstellerinformationen sind derzeit nicht verfügbar.
Benjamin S. Baumer is an associate professor in the Statistical & Data Sciences program at Smith College. He has been a practicing data scientist since 2004, when he became the first full-time statistical analyst for the New York Mets. Ben is a co-author of The Sabermetric Revolution and Analyzing Baseball Data with R. He received the 2019 Waller Education Award and the 2016 Significant Contributor Award from the Society for American Baseball Research.
Daniel T. Kaplan is the DeWitt Wallace emeritus professor of mathematics and computer science at Macalester College. He is the author of several textbooks on statistical modeling and statistical computing. Danny received the 2006 Macalester Excellence in Teaching award and the 2017 CAUSE Lifetime Achievement Award.
Nicholas J. Horton is Beitzel Professor of Technology and Society (Statistics and Data Science) at Amherst College. He is a Fellow of the ASA and the AAAS, co-chair of the National Academies Committee on Applied and Theoretical Statistics, recipient of a number of national teaching awards, author of a series of books on statistical computing, and actively involved in data science curriculum efforts to help students "think with data".
Daniel T. Kaplan is the DeWitt Wallace emeritus professor of mathematics and computer science at Macalester College. He is the author of several textbooks on statistical modeling and statistical computing. Danny received the 2006 Macalester Excellence in Teaching award and the 2017 CAUSE Lifetime Achievement Award.
Nicholas J. Horton is Beitzel Professor of Technology and Society (Statistics and Data Science) at Amherst College. He is a Fellow of the ASA and the AAAS, co-chair of the National Academies Committee on Applied and Theoretical Statistics, recipient of a number of national teaching awards, author of a series of books on statistical computing, and actively involved in data science curriculum efforts to help students "think with data".
I Part I: Introduction to Data Science. 1. Prologue: Why data science? 2.
Data visualization. 3. A grammar for graphics. 4. Data wrangling on one
table. 5. Data wrangling on multiple tables. 6. Tidy data. 7. Iteration. 8.
Data science ethics. II. Part II: Statistics and Modeling. 9. Statistical
foundations. 10. Predictive modeling. 11. Supervised learning. 12.
Unsupervised learning. 13. Simulation. III Part III: Topics in Data
Science. 14. Dynamic and customized data graphics. 15. Database querying
using SQL. 16. Database administration. 17. Working with spatial data.
18.Geospatial computations. 19. Text as data. 20. Network science. IV Part
IV: Appendices.
Data visualization. 3. A grammar for graphics. 4. Data wrangling on one
table. 5. Data wrangling on multiple tables. 6. Tidy data. 7. Iteration. 8.
Data science ethics. II. Part II: Statistics and Modeling. 9. Statistical
foundations. 10. Predictive modeling. 11. Supervised learning. 12.
Unsupervised learning. 13. Simulation. III Part III: Topics in Data
Science. 14. Dynamic and customized data graphics. 15. Database querying
using SQL. 16. Database administration. 17. Working with spatial data.
18.Geospatial computations. 19. Text as data. 20. Network science. IV Part
IV: Appendices.
I Part I: Introduction to Data Science. 1. Prologue: Why data science? 2.
Data visualization. 3. A grammar for graphics. 4. Data wrangling on one
table. 5. Data wrangling on multiple tables. 6. Tidy data. 7. Iteration. 8.
Data science ethics. II. Part II: Statistics and Modeling. 9. Statistical
foundations. 10. Predictive modeling. 11. Supervised learning. 12.
Unsupervised learning. 13. Simulation. III Part III: Topics in Data
Science. 14. Dynamic and customized data graphics. 15. Database querying
using SQL. 16. Database administration. 17. Working with spatial data.
18.Geospatial computations. 19. Text as data. 20. Network science. IV Part
IV: Appendices.
Data visualization. 3. A grammar for graphics. 4. Data wrangling on one
table. 5. Data wrangling on multiple tables. 6. Tidy data. 7. Iteration. 8.
Data science ethics. II. Part II: Statistics and Modeling. 9. Statistical
foundations. 10. Predictive modeling. 11. Supervised learning. 12.
Unsupervised learning. 13. Simulation. III Part III: Topics in Data
Science. 14. Dynamic and customized data graphics. 15. Database querying
using SQL. 16. Database administration. 17. Working with spatial data.
18.Geospatial computations. 19. Text as data. 20. Network science. IV Part
IV: Appendices.